The Art of Predicting the Beautiful Game: Unveiling Soccer Prediction Algorithms
Discover the fascinating science behind soccer prediction algorithms and how they revolutionize sports betting. Explore the key data points, model building process, and the future of this innovative technology.
Last Updated: 10/15/2023
The Popularity of Soccer and the Demand for Accurate Predictions
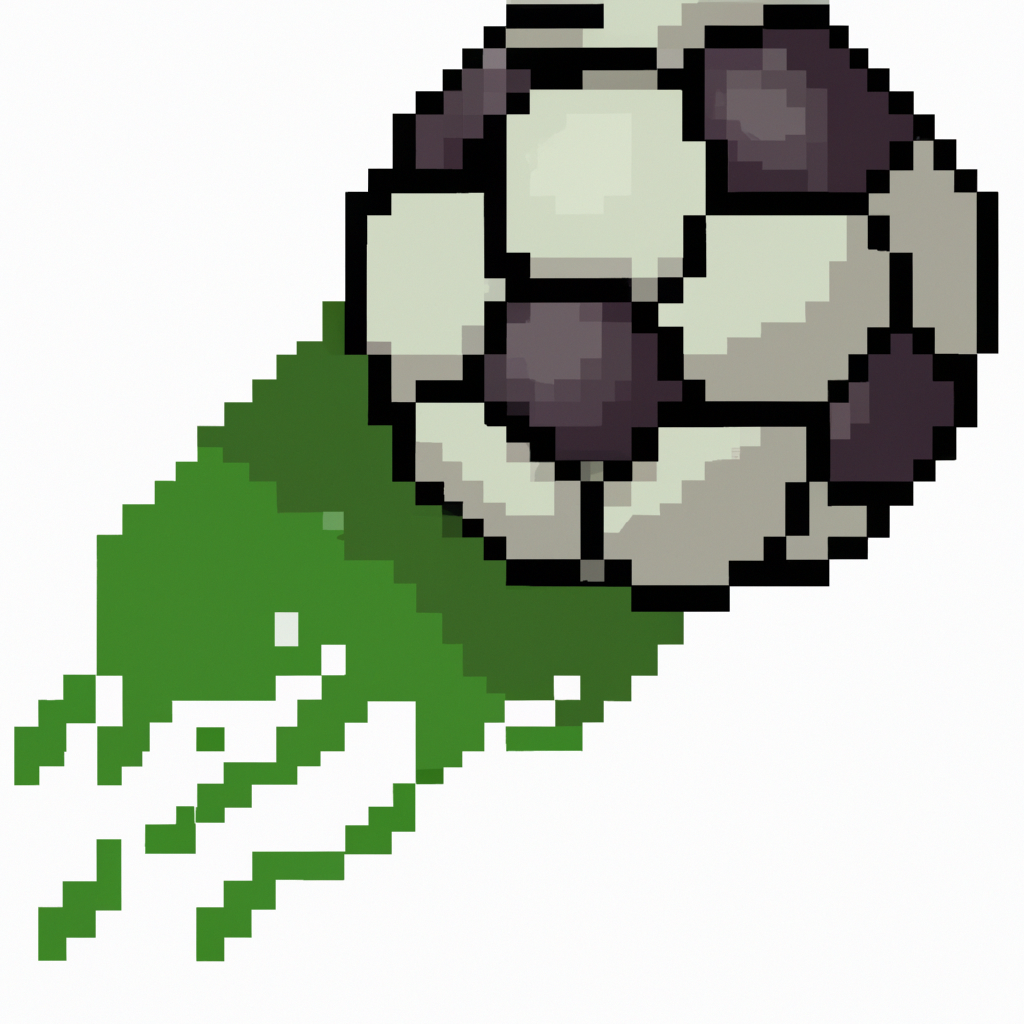
Exploring Soccer Prediction Algorithms and Their Impact on Sports Betting
Soccer, also known as football, is the most popular sport in the world, with billions of fans and spectators across the globe. The excitement and unpredictability of the game have captivated audiences for decades, leading to a growing interest in predicting match outcomes and player performances.
In recent years, there has been a surge in the demand for accurate soccer predictions. Sports enthusiasts, bettors, and even sportsbooks are constantly seeking reliable algorithms that can provide insights into the likely outcomes of soccer matches. This demand stems from the desire to make informed decisions when placing bets and to increase the chances of winning.
Soccer prediction algorithms use various statistical models, historical data, and machine learning techniques to analyze past performance, team dynamics, player statistics, and other relevant factors. These algorithms aim to generate predictions that can help bettors gain an edge and make more informed betting choices.
The potential impact of soccer prediction algorithms on sports betting is significant. By leveraging advanced algorithms, bettors can make more accurate predictions, leading to improved betting strategies and potentially higher winnings. Moreover, sportsbooks can also benefit from these algorithms by using them to set odds and lines more accurately, which can enhance their overall profitability.
However, it is important to note that no prediction algorithm can guarantee 100% accuracy. Soccer matches can be influenced by various factors such as injuries, weather conditions, and unexpected events that cannot be accounted for in algorithms. Nevertheless, soccer prediction algorithms can provide valuable insights and assist bettors in making more informed decisions.
In conclusion, the growing interest in soccer prediction algorithms reflects the desire for accurate predictions in the popular sport of soccer. These algorithms have the potential to significantly impact sports betting by providing valuable insights to bettors and sportsbooks alike. While they cannot guarantee perfect predictions, they can enhance the overall betting experience and potentially increase the chances of success.
In This Article..
- - The Popularity of Soccer and the Demand for Accurate Predictions
- - The Data Game: Exploring the key data points for soccer prediction algorithms
- - The Model Building Process: Unveiling the Inner Workings of Soccer Prediction Algorithms
- - Evaluating Performance: Assessing the effectiveness of soccer prediction algorithms
- - The Future of Soccer Prediction Algorithms
The Data Game: Exploring the key data points for soccer prediction algorithms
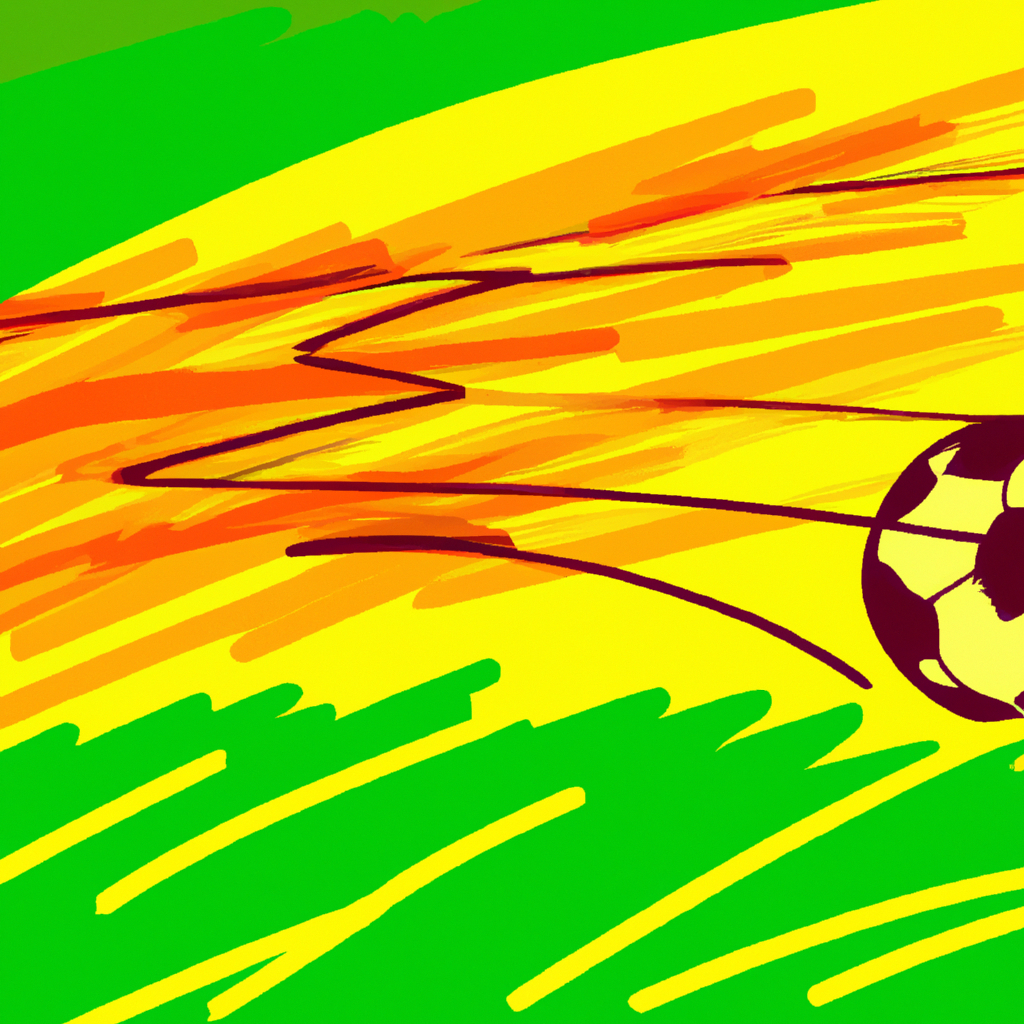
Importance of Historical Match Data
Historical match data is a crucial component in soccer prediction algorithms. By analyzing past match results, algorithms can identify trends, patterns, and historical performance of teams. This data provides valuable insights into team form, home and away performance, head-to-head records, and goal-scoring patterns. The analysis of historical match data allows algorithms to assess the probability of certain outcomes based on past occurrences.
For example, if a team has a strong home record and is playing against a team with a poor away record, algorithms can use historical match data to predict a higher likelihood of a home win. Moreover, the analysis of historical match data can also help in identifying specific strengths and weaknesses of teams, such as their defensive or attacking capabilities.
Overall, historical match data allows soccer prediction algorithms to make informed predictions by leveraging the patterns and trends observed in previous matches.
The Model Building Process: Unveiling the Inner Workings of Soccer Prediction Algorithms
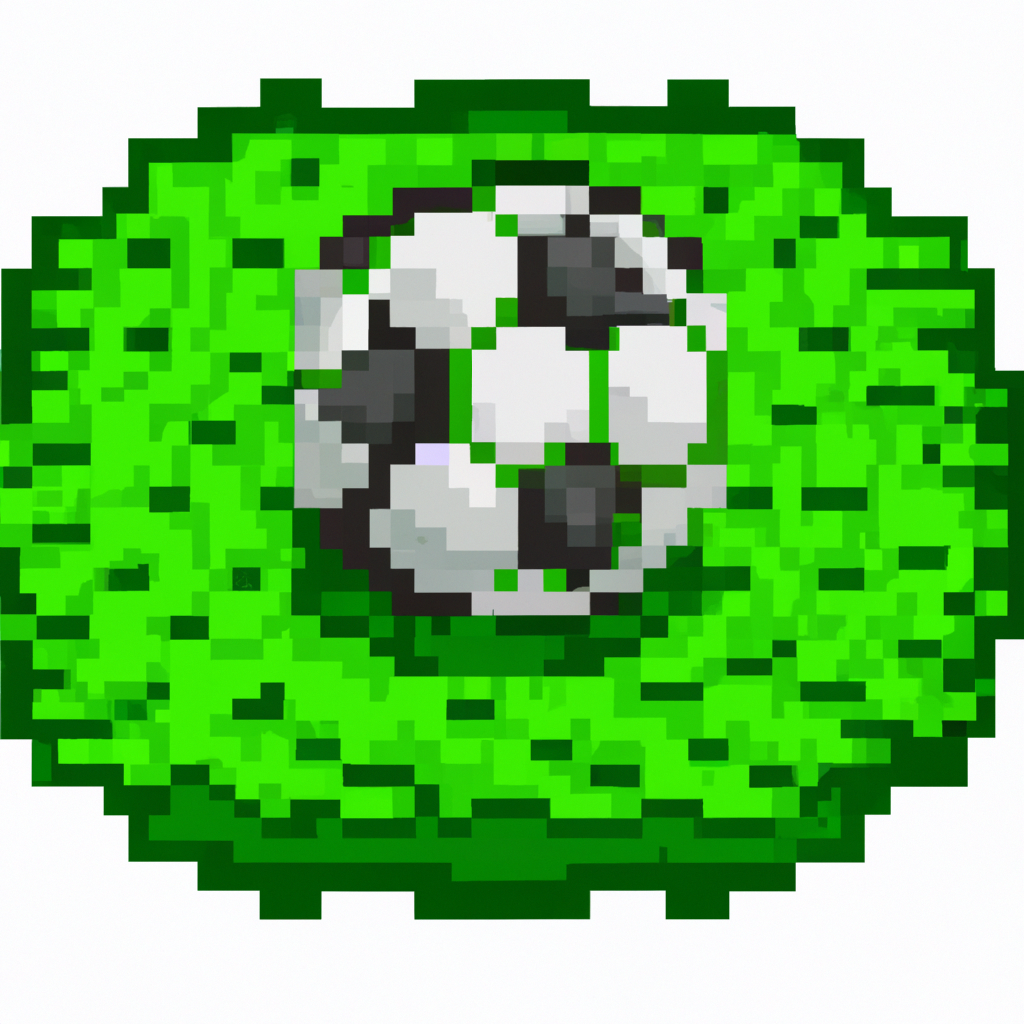
Exploring the Use of Machine Learning Techniques, Statistical Analysis, and Complex Algorithms
In the ever-evolving world of sports analytics, soccer prediction algorithms play a crucial role in providing accurate forecasts and insights. These algorithms leverage a combination of machine learning techniques, statistical analysis, and complex algorithms to build models that can accurately predict the outcome of soccer matches. Let's delve into the intricacies of the model building process and understand how these algorithms work.
Data Collection and Preprocessing
The first step in building a soccer prediction model is data collection. A vast amount of historical data is required, including match results, player and team statistics, weather conditions, and various other factors that can influence the outcome of a match.
Once the data is collected, it undergoes preprocessing to ensure its quality and consistency. This involves cleaning the data, handling missing values, and normalizing the features to bring them into a standard range. Data from different seasons and leagues are often combined to create a comprehensive dataset for analysis.
Feature Engineering
After preprocessing the data, the next step is feature engineering. This involves selecting and creating relevant features that can capture the important aspects of the game. Features can include team rankings, player performance metrics, previous match results, and various other factors that are believed to have an impact on the outcome.
Feature engineering requires domain expertise and a deep understanding of the game. It involves analyzing the data, exploring relationships between variables, and creating new features that can provide valuable insights.
Model Selection and Training
Once the features are defined, the next step is to select a suitable model for training. Various machine learning algorithms can be used, including logistic regression, support vector machines, random forests, and neural networks. The choice of model depends on the specific requirements of the prediction task and the characteristics of the data.
The selected model is then trained using the historical data. The dataset is split into training and testing sets, with the training set used to train the model and the testing set used to evaluate its performance. During the training process, the model learns the patterns and relationships in the data, adjusting its parameters to minimize prediction errors.
Model Evaluation and Validation
After the model is trained, it is evaluated and validated to assess its performance. Various evaluation metrics, such as accuracy, precision, recall, and F1 score, are used to measure the model's effectiveness. Additionally, techniques like cross-validation and holdout validation are employed to ensure the model's generalizability.
Model Refinement and Optimization
Building an accurate soccer prediction model is an iterative process. After evaluating the initial model, adjustments and refinements are made to improve its performance. This can involve tuning hyperparameters, adding or removing features, or trying different algorithms.
Sophisticated techniques like ensemble learning, which combines multiple models to make predictions, can be employed to further enhance the accuracy of the model.
Continuous Monitoring and Updates
Once the model is deployed, it is crucial to continuously monitor its performance and update it as new data becomes available. Soccer prediction models need to adapt to changing circumstances, such as player injuries, team dynamics, and other external factors that can influence match outcomes.
By continuously monitoring and updating the model, its predictive capabilities can be maintained and improved over time.
In conclusion, the model building process for soccer prediction algorithms involves data collection, preprocessing, feature engineering, model selection and training, evaluation and validation, refinement and optimization, and continuous monitoring and updates. Through a combination of machine learning techniques, statistical analysis, and complex algorithms, accurate soccer prediction models can be developed, providing valuable insights for sports analysts, bettors, and soccer enthusiasts.
Evaluating Performance: Assessing the effectiveness of soccer prediction algorithms
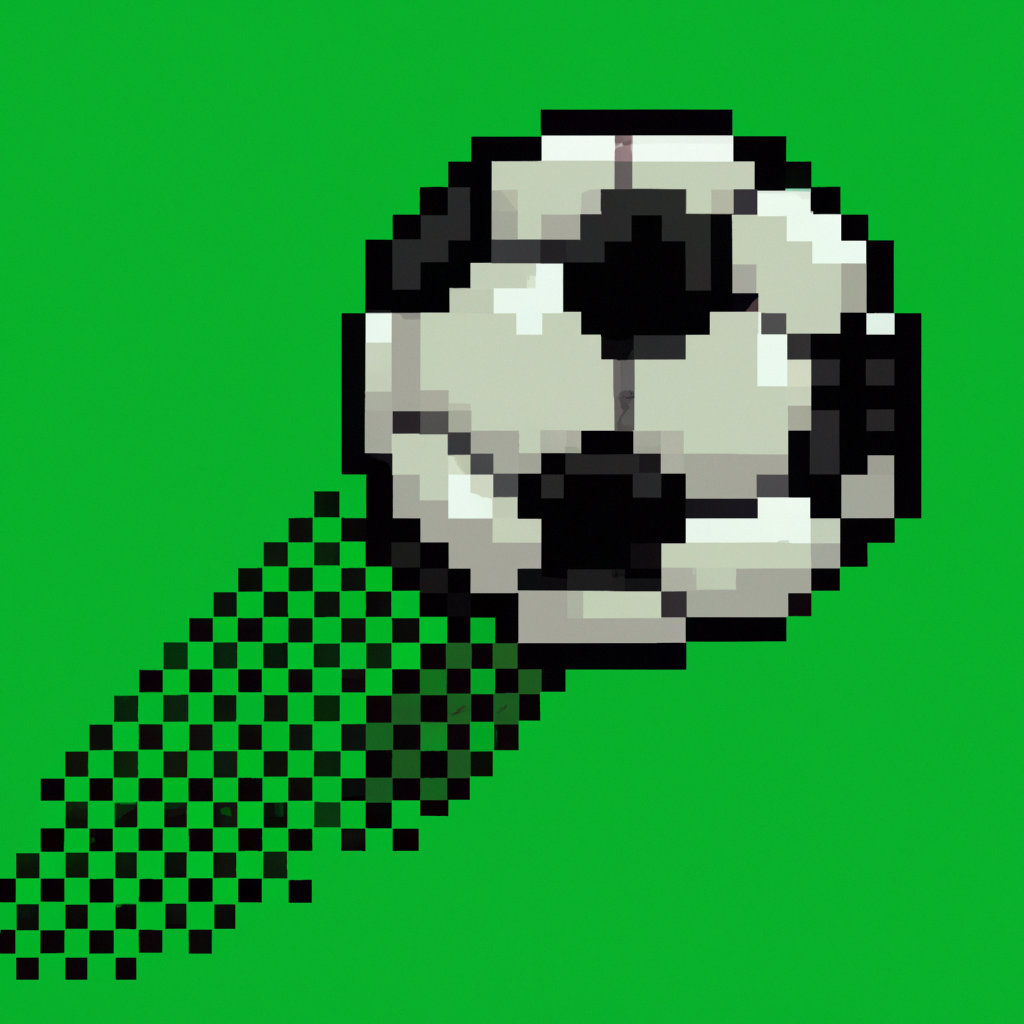
Metrics and Methods for Measurement
When evaluating the performance of soccer prediction algorithms, several metrics and methods are used to measure their accuracy and reliability. These metrics help assess the effectiveness of the algorithms and provide insights into their predictive capabilities.
-
Accuracy: Accuracy is a crucial metric for evaluating the performance of prediction algorithms. It measures the percentage of correct predictions made by the algorithm. Typically, accuracy is calculated by comparing the predicted outcomes with the actual results of soccer matches. A higher accuracy indicates a more reliable algorithm.
-
Precision and Recall: Precision and recall are metrics commonly used in classification problems, including soccer predictions. Precision measures the percentage of correctly predicted positive outcomes (e.g., correctly predicting a win). Recall, on the other hand, measures the percentage of actual positive outcomes that were correctly predicted. These metrics provide a deeper understanding of the algorithm's ability to identify specific outcomes.
-
F1 Score: The F1 score is a measure of the algorithm's accuracy that combines both precision and recall. It considers the balance between the two metrics and provides a single value to evaluate the algorithm's overall performance. The F1 score is particularly useful when there is an imbalance between positive and negative outcomes.
-
Mean Absolute Error (MAE): MAE is a metric used to measure the average deviation between the predicted and actual outcomes. In the context of soccer predictions, MAE quantifies the average difference between the predicted scores and the actual scores of matches. A lower MAE indicates higher accuracy and reliability.
-
Root Mean Squared Error (RMSE): RMSE is another metric used to assess the accuracy of prediction algorithms. It measures the square root of the average squared difference between the predicted and actual outcomes. RMSE is particularly useful when the prediction errors have a significant impact on the overall assessment.
-
Cross-Validation: Cross-validation is a method used to evaluate the performance of prediction algorithms by splitting the available data into multiple subsets. The algorithm is trained on a subset of the data and tested on the remaining subset. This process is repeated several times, and the average performance across all iterations is used to assess the algorithm's reliability.
-
Confusion Matrix: A confusion matrix is a table that summarizes the performance of a classification algorithm. It provides a detailed breakdown of the true positives, true negatives, false positives, and false negatives. This matrix helps evaluate the algorithm's ability to correctly predict different outcomes.
These metrics and methods provide a comprehensive framework for evaluating the effectiveness of soccer prediction algorithms. By considering multiple dimensions of performance, it is possible to assess the accuracy and reliability of these algorithms and make informed decisions based on their predictions.
The Future of Soccer Prediction Algorithms
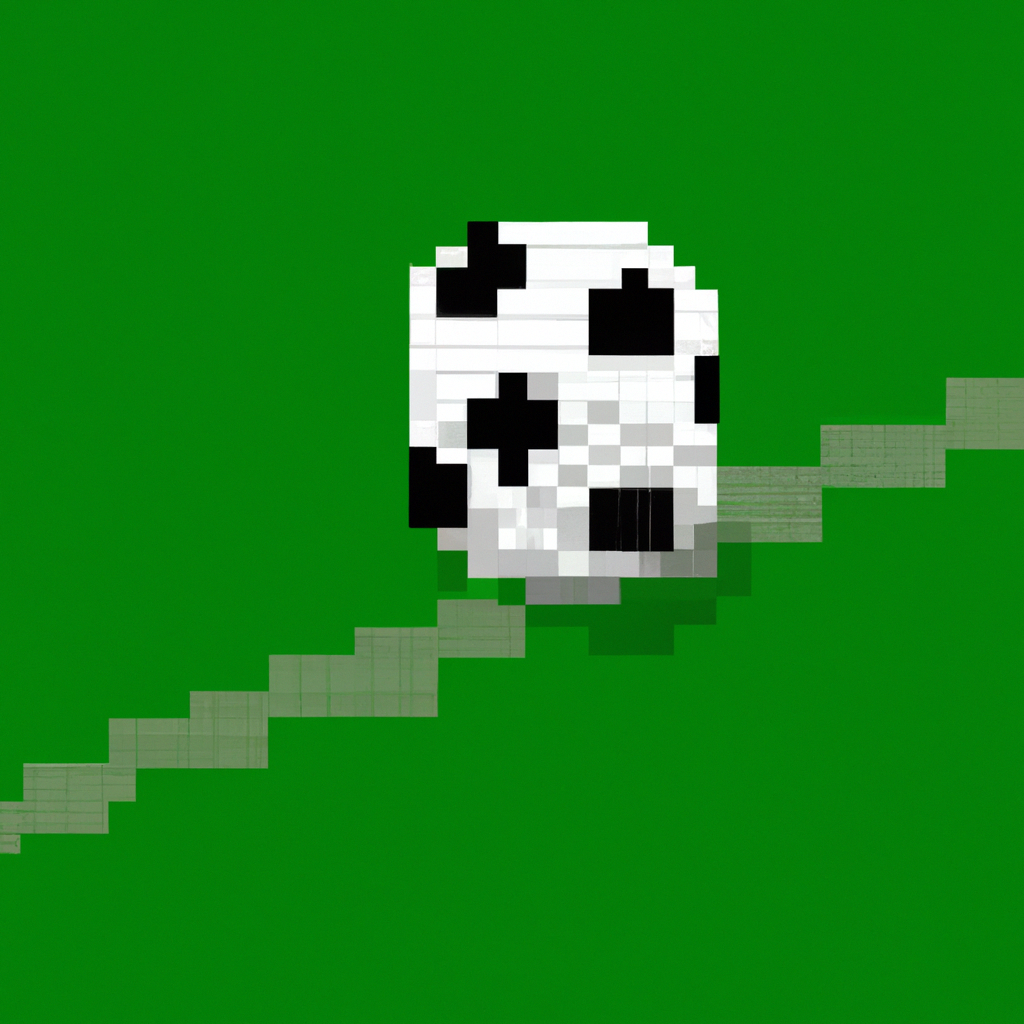
Advancements in Technology and Data Analytics
Soccer prediction algorithms have come a long way in recent years, but the future holds even more exciting potential for advancements in technology and data analytics. With the rapid growth of machine learning and artificial intelligence, soccer prediction algorithms can become even more accurate and reliable.
One potential advancement is the use of advanced statistical models that can analyze vast amounts of data. These models can take into account various factors such as player performance, team tactics, weather conditions, and historical data to make more precise predictions. By leveraging big data and sophisticated algorithms, these models can uncover patterns and trends that humans may overlook.
Another area of advancement is the incorporation of real-time data. With the advent of sensors and tracking technologies, it is now possible to collect real-time data during soccer matches. This data includes information about player movements, ball trajectories, and team formations. By integrating this real-time data into prediction algorithms, analysts can get a more accurate picture of the current state of a game and adjust their predictions accordingly.
Furthermore, advancements in technology can improve the accessibility and usability of soccer prediction algorithms. Mobile apps and online platforms can provide users with instant access to predictions, analysis, and live updates. This allows fans and bettors to make informed decisions and stay engaged with the game.
In conclusion, the future of soccer prediction algorithms looks promising. Advancements in technology and data analytics can enhance the accuracy and reliability of predictions. With the integration of advanced statistical models, real-time data, and improved accessibility, soccer prediction algorithms can provide valuable insights to fans, bettors, and teams alike.
Related Content
- Best Side Hustle Tools
Best Free Tools for Side Hustlers - #1 will surprise you
FREELaunch your side hustle in seconds with these FREE tools.
- Dumpster Fire Jobs
Get a Job When No One is Hiring
USEFULPractical Tips for searching for a job in a **** economy.